
Startup Projects
Marine Conservation

Project “liquet”
Marine Ecology Analysis
Artificial Intelligence (AI) and Machine Learning (ML) based systems used to analyze underwater video footage of coral reef ecosystems and deliver predictive analysis on the health and wellness of the reef ecosystem. Population analysis, migration patterns, historical comparisons, breeding and growth patterns analysis, species identification, and desegregation analysis are but a few of the platforms' tools.
Currently under development is a self-contained solar-powered hardware module used to constantly stream remote video data. This hardware module coupled with the analytics platform will provide scientists with invaluable datasets and insight into one of the world's most precious resources.
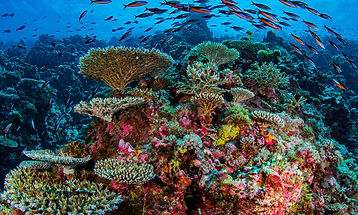

Project “pistris”
Preventive Capture Targeting
Thousands of shark and other endangered large sea animals are killed each year due to shark nets. Due to policies in many countries, the practice of deploying shark nets to protect beach-goers from attacks, will continue to be the preferred approach.
Project "pistris" is an AI-enabled auto pilot drone technology that is used to patrol beaches where shark nets are deployed in order to identify approaching sharks or signs of other large marine animal activity. Once identified and assessed as being in danger of entanglement, the drone deploys repellent technologies appropriate for the specific species of marine animal.
Through the use of cybernetic modelling, much of the behavior of each marine species can be predicted and thus treated with different repellent technologies.
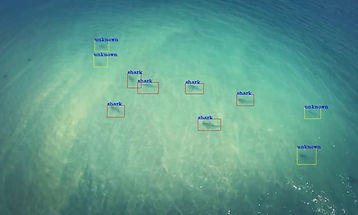

Project “quisquiliaq”
Refuse and Debris Predictive Analysis
The world's beaches and river systems are clogged with refuse and debris. Project "quisquiliaq" is focused on identifying existing refuse and debris patterns. Through the use of Machine Learning (ML) and cybernetic models, the Project "quisquiliaq" team is focused on providing detailed predictive refuse and debris mapping.
Data is collected through the use of automated drones that transverse coastlines and river-ways collecting visual data. This data is then analyzed to identify the type, density, deposit rates, volume, time-at-site, decomposition rate, and redistribution patterns.


Project “exesa”
Coastline Erosion Modelling
Coastlines around the world are constantly changing, and some of these changes are part of a natural cycle, with man-made impact increasingly having a dramatic effect. These changes can cause extreme disruption and are very problematic for coastal populations, not to mention the massive economic impact.
Project "exesa" is a Deep Learning (DL) system built on cybernetic models that helps identify and predict coastline and estuary erosion patterns. Remote sensing is used to gather data over time and build predictive models. These models take into account many elements of the natural and man made systems.
Topographical, geological, oceanographic, meteorologic, and climatological data sources are used to build detailed predictive models utilizing the power of Artificial Intelligence (AI), Machine Learning (ML), and Deep Learning.
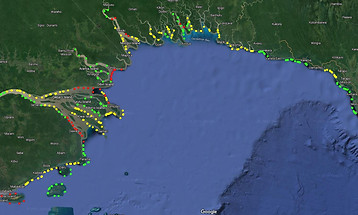